Séminaire NUMEV “Modélisation basée sur des données et prédiction de séries temporelles grâce à l’équation de Langevin généralisée”
Cet évènement est passé !
Les Séminaires NUMEV sont ouverts à un large public d’étudiants, étudiantes, chercheurs et chercheuses de toutes disciplines, qui souhaitent en savoir plus sur les domaines de recherche actuels de la communauté NUMEV-MIPS (Mathématiques, Informatique, Physique et Systèmes) ou sur les possibilités de développer ses compétences et savoir-faire.
Par Roland Netz, Department of Physics, Free University Berlin
La plupart des systèmes d’intérêt scientifique sont des systèmes à N corps en interaction. On décrit généralement leur cinétique en termes de coordonnées de réaction de faible dimension, qui sont généralement influencées par l’ensemble du système. La dynamique d’une telle coordonnée de réaction est régie par l’équation de Langevin généralisée (ELG), une équation stochastique intégro-différentielle, et implique une fonction de mémoire, qui décrit comment cette dynamique dépend des valeurs précédentes de la coordonnée de réaction. La GLE est donc une description systématique non markovienne de la dynamique d’un système en termes de variables à large granularité (coarse-grained). Nous avons récemment introduit un nouveau schéma de projection hybride qui permet d’extraire les paramètres GLE à partir de données de séries temporelles, sous une forme qui convient aux traitements analytiques et numériques. Au cours de cet exposé, j’aborderai quelques exemples dans lesquels l’EGL peut être utilisée pour interpréter et modéliser des données dans différents domaines scientifiques.
« Data based modeling and time series prediction using the generalized Langevin equation »
Roland Netz, Department of Physics, Free University Berlin
Abstract
Most systems of scientific interest are interacting many-body systems. One typically describes their kinetics in terms of a low-dimensional reaction coordinate, which in general is influenced by the entire system. The dynamics of such a reaction coordinate is governed by the generalized Langevin equation (GLE), an integro-differential stochastic equation, and involves a memory function, which describes how the dynamics depends on previous values of the reaction coordinate. The GLE is thus an systematic non-Markovian description of the dynamics of a system in terms of coarse-grained variables. We have recently introduced a novel hybrid projection scheme that allows to extract the GLE parameters from time series data in a form that is convenient for analytic and numerical treatments. In the talk I discuss a few examples where the GLE can be used to interpret and model data in different fields of science.
Protein-folding kinetics is typically described as Markovian (i.e., memoryless) diffusion in a one-dimensional free energy landscape, governed by an instantaneous friction coefficient that is fitted to reproduce experimental or simulated folding times. According to this view, the folding time is dominated by the exponential of the folding free energy barrier, the Arrhenius factor, where the friction coefficient only sets the pre-exponential time scale and plays a subordinate role. By analysis of large-scale molecular-dynamics simulation trajectories of fast-folding proteins from the Shaw group using the special-purpose computer ANTON, it is demonstrated that the friction characterizing protein folding exhibits significant memory with a decay time that is of the same order as the folding and unfolding times. Non-Markovian modeling not only reproduces simulations accurately but also demonstrates that memory friction effects lead to anomalous and drastically modified protein kinetics. For the set of proteins for which simulations are available, it is shown that the folding and unfolding times are not dominated by the free-energy barrier but rather by the non-Markovian friction.
Memory effects are also present for non-equilibrium systems. Using an appropriate non-equilibrium formulation of the GLE, it is demonstrated that the motion of living organisms is characterized by memory friction, which allows to characterize internal feedback loops of such organisms and to classify and sort individual organisms The GLE can be even used to predict complex phenomena such as weather data.
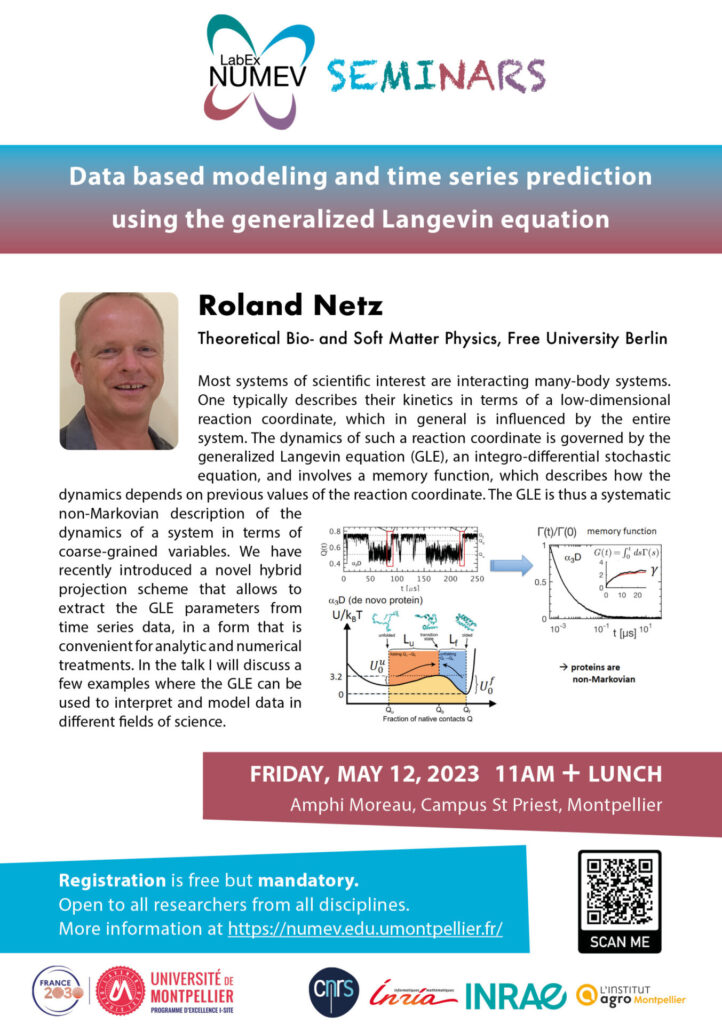
Recevoir un récapitulatif de l’agenda de l’UM chaque semaine
* En renseignant votre mail vous acceptez de recevoir chaque semaine le récapitulatif de l’agenda de l’UM par courrier électronique et vous prenez connaissance de notre politique de confidentialité. Vous pouvez vous désinscrire à tout moment à l’aide du lien de désinscription ou en nous contactant par mail.